當下最熱門的職業(yè):數(shù)據(jù)翻譯師
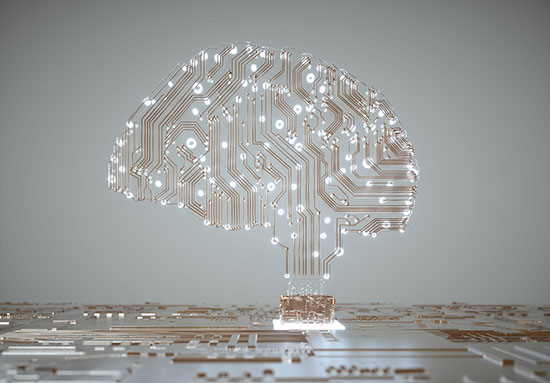
數(shù)據(jù)翻譯師這個職業(yè)存在著巨大的需求(而且還在不斷增長),這項工作要求人們將數(shù)據(jù)科學轉(zhuǎn)化為能夠推動變革或解決問題的行動方案,。 美國萬通保險這家價值300億美元的人壽保險公司遇到了一個問題,。當時是在2013年,與其他的險企一樣,,該公司因為騙保而備感苦惱,。美國聯(lián)邦調(diào)查局估計,騙保每年讓美國保險行業(yè)(以及投保人)虧損400億美元,。萬通保險的首席技術策略和數(shù)據(jù)科學負責人西爾斯·梅里特說:“我們不得不大幅改善我們對騙保的實時識別能力,?!?/p> 萬通保險在公司數(shù)據(jù)科學家和其業(yè)務經(jīng)理之間發(fā)起了創(chuàng)新的合作模式,并創(chuàng)建了產(chǎn)品經(jīng)理這個新職位,。產(chǎn)品經(jīng)理將在數(shù)據(jù)分析師與負責公司不同業(yè)務的日常決策者之間擔任溝通工作,。一開始,產(chǎn)品經(jīng)理將從各個部門——人壽,、傷殘,、長期護理等等——搜集信息,然后向數(shù)據(jù)分析師解釋應該通過哪些手段來發(fā)現(xiàn)和打擊每個領域的騙保行為,。數(shù)據(jù)科學家然后選擇和定制相關數(shù)據(jù),,然后產(chǎn)品經(jīng)理幫助業(yè)務經(jīng)理將其轉(zhuǎn)化為具體的防騙保舉措。 如今,,萬通保險在全企業(yè)采取了這一舉措,,不僅僅局限于騙保識別。梅里特說:“它適用于每一個流程和每一個業(yè)務線,,從營銷,、承保一直到理賠。結(jié)果真的非常有效,?!?/p> 數(shù)據(jù)科學家和業(yè)務經(jīng)理之間的合作發(fā)現(xiàn)了效率低下的領域以及業(yè)務增長的新途徑。梅里特指出,,這一舉措將萬通保險的營收和利潤提升了“數(shù)千萬美元”,,而且產(chǎn)品經(jīng)理“對于實現(xiàn)這一業(yè)績至關重要?!?/p> 數(shù)據(jù)正在迅猛地滲透各個領域,,但經(jīng)理和高管對于數(shù)據(jù)的認識并沒有跟上其節(jié)奏?!豆鹕虡I(yè)評論》指出,,這也是為什么數(shù)據(jù)翻譯師成為了一個“必須設置的分析職務”。即便你以前從未聽說過數(shù)據(jù)翻譯師這一術語——例如萬通保險的產(chǎn)品經(jīng)理,,但他可能已經(jīng)成為了你的合作伙伴,。毫無疑問的是,那些擅長在現(xiàn)實生活中解讀數(shù)據(jù),,并在實際中加以運用的人才當前已經(jīng)成為了備受追捧的商品,。麥肯錫全球研究所預計,到2026年,,數(shù)據(jù)翻譯師在美國的需求就將達到200萬至400萬,。 當前,聘請數(shù)據(jù)翻譯師并不容易。部分原因在于這項工作要求雇員擁有獨特的組合技能,,通常包括扎實的數(shù)據(jù)科學以及將復雜理念簡化為明確,、實用選項的才能。全球云軟件巨頭Infor旗下公司Birst的產(chǎn)品策略副總裁布拉德·斯蒂爾威爾稱,,這些人如此之稀缺,,以至于他們“屬于招聘者所稱的‘獨角獸’門類?!?/p> 斯蒂爾威爾在他18年的職業(yè)生涯中招聘了多名翻譯師,。他指出,盡管人工智能可被用于在某些問題上為業(yè)務經(jīng)理提供建議,,并回答他們與數(shù)據(jù)相關的某些問題,,但它依然無法取代人類。斯蒂爾威爾說:“這項工作存在一定的藝術性,。商業(yè)決策通常必須依據(jù)不完整的信息,,并依靠直覺和創(chuàng)意,而且決策的時間也很緊張,。因此理想的翻譯師需要同時擅長左腦和右腦思維,。” 斯蒂爾威爾說,,這也是為什么“在團隊中與數(shù)據(jù)分析師緊密合作的文科畢業(yè)生通常會成為翻譯大師的原因,。一些攻讀歷史專業(yè)的人可能并不知道如何進行線性數(shù)據(jù)調(diào)度,但他們深知如何通過研究歷史數(shù)據(jù)來發(fā)現(xiàn)規(guī)律,,并推斷其可能的結(jié)果?!?/p> 似乎同時具備數(shù)學天賦和溝通才能的人才倒也不算太稀缺,,然而,最為有效的翻譯師還具備另一項能力:全面了解所從事的業(yè)務,。如果沒有這個層面的信息,,他們就無法理解業(yè)務經(jīng)理需要從數(shù)據(jù)中搜集的內(nèi)容以及原因。擁有以上三方面要素的人才的確是少之又少,,所以這里就不光是獨角獸那么簡單,,而是帶波爾卡圓點的粉色獨角獸。因此,,很多公司已經(jīng)放棄嘗試從外部招聘翻譯師,,而是在公司內(nèi)部培養(yǎng)。例如,,麥肯錫在數(shù)年前便推出了內(nèi)部培養(yǎng)機構,,如今每年能夠提供約1000名數(shù)據(jù)翻譯師。 萬通保險也走上了同樣的道路,。這家險企在2014年與其馬薩諸塞州西部的五家大學合作,,共同創(chuàng)建了其數(shù)據(jù)科學開發(fā)項目(DSDP),。該項目的學生會先在包括史密斯學院、芒特·霍利奧克學院和馬薩諸塞大學安姆斯特分校在內(nèi)的幾所學校學習為期三年的數(shù)據(jù)密集型課程,,然后加入萬通保險擔任初級數(shù)據(jù)科學家,,同時就讀數(shù)據(jù)科學研究生課程。新招聘員工會與高級雇員并肩作戰(zhàn),,將數(shù)據(jù)運用至萬通保險經(jīng)理所面臨的日?,F(xiàn)實世界中的商業(yè)挑戰(zhàn)。 DSDP項目提供數(shù)據(jù)科學和數(shù)據(jù)翻譯方面的培訓,,在任何時候都只有約20名學生和少數(shù)研究生就讀,。負責該項目的梅里特說:“算法只能告訴哪些是你可以解決的商業(yè)問題。但人類的判斷和直覺則要強大的多,,它們能夠告訴你應該去解決哪些問題,。” 數(shù)據(jù)背后的故事 在未來,,我們所有人可能都得學一些數(shù)據(jù)翻譯技能,。Salesforce的首席執(zhí)行官馬克·貝尼歐夫在南希·度爾特的新書《數(shù)據(jù)故事:通過故事來解釋數(shù)據(jù)和激勵行動》(DataStory: Explain Data and Inspire Action Through Story)中說:“我們需要新一代的高管能夠了解如何在茫茫數(shù)據(jù)中進行管理和領導,。而且我們還需要新一代的雇員能夠幫助我們圍繞這些數(shù)據(jù)來組織和構建我們的業(yè)務,。” 換句話說,,幾乎所有的工作都得使用大多數(shù)雇員從未用過或需要過的數(shù)據(jù)翻譯技能,。 當然,一些人生來就有能力讓枯燥的事實變得豐富多彩,、引人入勝,,尤其是我們中間的那些健談者。硅谷傳播公司Duarte, Inc.的負責人南?!ざ葼柼卣J為,,講故事——將蒼白、枯燥的數(shù)據(jù)轉(zhuǎn)化為其受眾能夠輕易理解和記住的生動故事——要比大多數(shù)其他翻譯技巧更有效,,由其是在勸說人們開展某種具體行動的時候,。 這是因為,受大腦構造的影響,,人類明顯更渴望聽到帶有情節(jié)的故事,,其中包括開始、過程和結(jié)局,。她說:“MRI圖像展示,,相對于對數(shù)據(jù)的反應,大腦對基于數(shù)據(jù)的故事的反應要強烈得多?!彼€說,,數(shù)據(jù)“在沒有人類經(jīng)驗和判斷的情況下毫無用處。如果我們僅依靠機器進行決策,,那么這些決策都將是錯誤的,。”(財富中文網(wǎng)) 譯者:馮豐 審校:夏林 |
There is a tremendous (and growing) need for data translators, a job that requires people to be able to interpret data science into actions that drive change or fix problems. MassMutual, a $30 billion per year life insurance company, had a problem. It was 2013 and, along with the rest of the insurance industry, it was bedeviled by fraud. According to FBI estimates, fraud sets the U.S. insurance industry (and policyholders) back by $40 billion a year. “We had to get much better at detecting fraud in real time,” says Sears Merritt, MassMutual’s chief of technology strategy and data science. So MassMutual launched an innovative collaboration between the company’s data scientists and its line managers. They created a new role, product managers, who act as translators between the data analysts and the day-to-day decision-makers who run the company’s various lines of business. At the outset, the product managers gathered information from each department—life, disability, long-term care, and so on—and explained to data analysts exactly what was needed to spot, and thwart, fraud in each area. The data scientists then culled and customized the relevant numbers, which the product managers helped line managers translate into specific antifraud moves. Now MassMutual relies on this approach companywide, far beyond fraud detection. It works “in every process, in every line of business from marketing to underwriting to claims,” says Merritt. “The results have been really impactful.” The collaboration between data scientists and line managers has pinpointed inefficiencies and identified new pathways to growth. That has boosted MassMutual’s revenues and profits by “tens of millions of dollars,” says Merritt, and the product managers “have been crucial to making it happen.” Data is proliferating at warp speed, but data literacy among managers and executives hasn’t caught up. That’s why data translators have, according to the Harvard Business Review, become a “must-have analytics role.” Even if you’ve never heard the term data translator, you may already be working with one. Because they go by so many different titles—like MassMutual’s product managers—no one knows how many translators exist right now. But there’s no doubt that people who are adept at interpreting data for practical use in the real world are a hot commodity. By 2026, the McKinsey Global Institute predicts that there could be a demand for 2 million to 4 million translators in the U.S. alone. At the moment, hiring translators isn’t easy. That’s partly because the job requires a unique combination of skills, usually including both a strong grounding in data science and a talent for boiling complex ideas down to clear, practical choices. They’re so rare that translators “belong to a category recruiters call ‘unicorns’,” notes Brad Stillwell, vice president of product strategy at Birst, a unit of global cloud software giant Infor. Stillwell has hired a number of translators in his 18-year career. He notes that though artificial intelligence can be used to advise line managers on some issues and answer some of their data-related questions, it can’t replace humans. “There is still an art to it,” Stillwell says. “Business decisions often have to be made based on incomplete information, using intuition and creativity, and without much time. So the ideal translator is equally adept at both left- and right-brain thinking.” That’s why “l(fā)iberal arts graduates, collaborating closely on a team with data analysts, often make great translators,” Stillwell says. “Someone who majored in history may not know how to do a linear data progression, but they often do know, from studying historical data, how to spot patterns and infer where the data might lead.” As if a mathematical bent and a knack for communications together weren’t scarce enough, the most effective translators bring with them one more thing: a thorough knowledge of the business they’re working in. Without that level of information, they won’t be able to understand what line managers need to glean from the data and why. People with this trifecta of talents are so scarce—so not just unicorns but pink unicorns with purple polka dots—that many companies have given up trying to hire translators from outside and are training them in-house instead. McKinsey, for instance, launched its own internal academy a few years ago, which now turns out about 1,000 data translators annually. MassMutual has taken this route, too. The insurer launched its Data Science Development Program (DSDP) in 2014, in partnership with five colleges near its western Massachusetts headquarters. After a data-intensive three-year curriculum at the schools, including Smith, Mount Holyoke, and UMass Amherst, graduates join MassMutual as junior data scientists, while attending grad school in data science at the same time. The new hires work alongside senior colleagues on applying data to the everyday, real-life business challenges that MassMutual line managers face. The DSDP program, which has about 20 people and a handful of grad students at any given time, offers training in both data science and translation. “Algorithms can only tell you what business problems you can solve,” says Sears Merritt, who runs the program. “But human judgment and intuition can go way beyond that, and tell you what problems you should solve.” The story behind data In the years ahead, we may all need to learn some data translation skills. “We need a new generation of executives who understand how to manage and lead through data,” says Salesforce CEO Marc Benioff in Nancy Duarte’s new book, DataStory: Explain Data and Inspire Action Through Story. “And we also need a new generation of employees who are able to help us organize and structure our businesses around that data.” Just about every job, in other words, will call for data-translation skills that most employees haven’t used, or needed, yet. Of course, some people have a natural ability to render dry facts colorful and interesting, especially the born raconteurs among us. Nancy Duarte, head of Silicon Valley communications firm Duarte, Inc., believes that storytelling—turning plain, eye-glazing heaps of data into a vivid tale its audience can easily grasp and remember—works better than most other translation techniques, especially when it comes to persuading people to take a specific course of action. That’s because the human brain is apparently hardwired to crave narratives with plots that include a beginning, a middle, and an end. “MRI images show that telling a story based on data lights up the human brain in a way that data alone just can’t match,” she says, adding that data is “useless without human experience and judgment. If we relied solely on machines to make decisions, they’d all be the wrong ones.” |