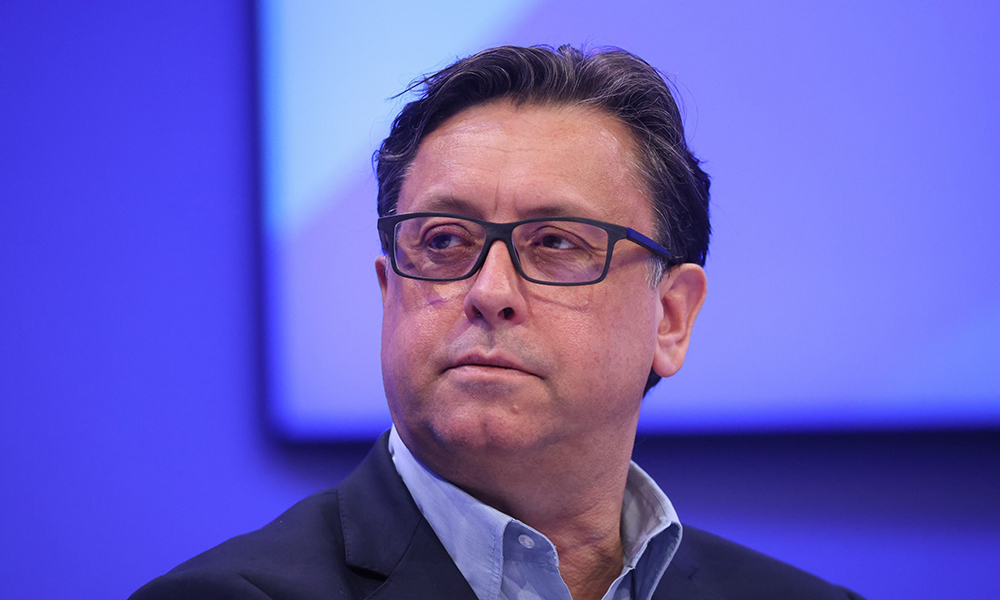
在這樣一個(gè)經(jīng)濟(jì)充滿不確定性、利率高企,、人才短缺,、資本成本不斷上升的時(shí)代,人工智能(AI)已經(jīng)成為許多行業(yè),、政府部門和學(xué)術(shù)機(jī)構(gòu)最關(guān)心的話題,。雖然還有很多問題亟待醫(yī)療保健行業(yè)解決,但我們有理由相信,,隨著人工智能技術(shù)的不斷發(fā)展,,藥物發(fā)現(xiàn)的黃金時(shí)代即將到來,而這將從根本上改變醫(yī)學(xué)領(lǐng)域的整體面貌,。
人工智能已經(jīng)開始在制藥研發(fā)(R&D)領(lǐng)域發(fā)揮作用,。在人工智能與數(shù)據(jù)分析的加持之下,業(yè)界實(shí)現(xiàn)了許多突破,,比如現(xiàn)在我們已經(jīng)能夠預(yù)測(cè)患者反應(yīng),,臨床試驗(yàn)的成功率也有所提升,并且已經(jīng)開始為患者確定個(gè)性化的治療方案,。借助人工智能,,我們解決了許多技術(shù)難題,解鎖了許多以前“無藥可及”的靶點(diǎn),,為目前沒有治療選擇的患者帶來新的治療方法,。
在賽諾菲(Sanofi)的藥物發(fā)現(xiàn)和開發(fā)工作中,人工智能技術(shù)已經(jīng)開始發(fā)揮重要作用,。在小分子藥物發(fā)現(xiàn)方面,,我們關(guān)鍵人工智能模型的預(yù)測(cè)準(zhǔn)確率已經(jīng)達(dá)到80%以上,而且通過主動(dòng)學(xué)習(xí),,這些模型的準(zhǔn)確率還在不斷提高,。我們?cè)诖_定疾病靶點(diǎn)時(shí),有90%借助了單細(xì)胞基因組學(xué)方面的知識(shí),,在推進(jìn)小分子項(xiàng)目時(shí),,有75%借助了人工智能與機(jī)器學(xué)習(xí)(ML)化合物設(shè)計(jì),。我們還創(chuàng)造了虛擬患者,來推動(dòng)硅基臨床試驗(yàn),,最后,,我們利用基于基因組學(xué)的精準(zhǔn)醫(yī)療技術(shù),協(xié)助開展患者分層工作,。
我們使用先進(jìn)的主動(dòng)學(xué)習(xí)方法,,持續(xù)改進(jìn)人工智能模型訓(xùn)練方式,不斷減少模型訓(xùn)練所需的數(shù)據(jù),。人工智能學(xué)習(xí)凸顯出了關(guān)鍵結(jié)構(gòu)要素在引導(dǎo)設(shè)計(jì)周期方面的作用,,可以縮短設(shè)計(jì)周期,降低設(shè)計(jì)成本,,并提高設(shè)計(jì)出新分子的成功率,。我們正在將臨床試驗(yàn)的數(shù)量增加50%,根據(jù)現(xiàn)有數(shù)據(jù),,我們?cè)谘兴幬锏膬r(jià)值在2019年至2023年間翻了兩番,。
我們始終堅(jiān)持與創(chuàng)新生態(tài)保持聯(lián)系,采取“無邊界”藥物研發(fā)戰(zhàn)略,。我們25%的項(xiàng)目要與合作伙伴合作完成,,按照每種臨床候選藥物所花費(fèi)的資金來衡量,這種做法使我們的研究效率提高了一倍,,并讓我們能夠達(dá)到首次人體實(shí)驗(yàn)階段的藥物數(shù)量增加了一倍,。
此外,我們的運(yùn)作方式也在發(fā)生深刻變化,?!皼Q策”不再是一種年度回顧性報(bào)告工作,而是變?yōu)榱藙?dòng)態(tài)的前瞻性決策方法,,將戰(zhàn)略選擇與業(yè)務(wù)決策聯(lián)系起來,,努力強(qiáng)化我們的反饋路徑。
我們現(xiàn)在顯然已經(jīng)站到了醫(yī)學(xué)發(fā)現(xiàn)大發(fā)展的十字路口,,但是,,制造企業(yè)要想充分發(fā)揮人工智能的優(yōu)勢(shì),充分利用其潛力,,我們還要克服若干具有深遠(yuǎn)影響的難題,。
監(jiān)管問題
不同地區(qū)在監(jiān)管方面存在差異,這種差異會(huì)限制人工智能的應(yīng)用領(lǐng)域和標(biāo)準(zhǔn),,同時(shí)還會(huì)對(duì)“高風(fēng)險(xiǎn)應(yīng)用”的定義產(chǎn)生影響,。
對(duì)數(shù)據(jù)質(zhì)量、安全性、隱私和可信度的擔(dān)憂可能會(huì)減緩人工智能技術(shù)的應(yīng)用速度?,F(xiàn)在已經(jīng)出現(xiàn)了一些以幫助企業(yè)實(shí)現(xiàn)自我監(jiān)管為目的的聯(lián)盟和組織,。
隨著許多企業(yè)開始全面應(yīng)用人工智能技術(shù),防止漏洞出現(xiàn)就成了相關(guān)企業(yè)面臨的重要任務(wù),,而要達(dá)成這一目標(biāo),,就必須打造強(qiáng)大的數(shù)據(jù)基礎(chǔ)和治理機(jī)制。
限價(jià)的意外影響
新推出的定價(jià)政策可能會(huì)帶來一些意想不到的后果,,甚至可能降低投資人對(duì)一些很有希望的候選研發(fā)項(xiàng)目的投資意愿。例如,,《通脹削減法案》(Inflation Reduction Act)里就包含一些人口中所謂的“藥丸懲罰”條款,,相關(guān)條款規(guī)定,在小分子藥物獲批后9年,、生物制劑獲批后13年,,政府能夠?qū)π卵兴幬镞M(jìn)行限價(jià)??梢哉f,,這種規(guī)定從根上打消了藥企為老藥尋求新突破和新用途的動(dòng)力。還可能導(dǎo)致藥企加大對(duì)生物制劑的投資,,減少對(duì)小分子藥物的投資,。
生物制劑和小分子藥物具有同等價(jià)值。小分子藥物可以口服,,對(duì)許多患者而言更為方便,,在許多疾病的治療上也發(fā)揮著至關(guān)重要的作用。
生物技術(shù)初創(chuàng)企業(yè)獲得資金的途徑
生物技術(shù)初創(chuàng)企業(yè)擁有良好的創(chuàng)新環(huán)境,,是大型制藥公司研發(fā)工作的有益補(bǔ)充,。二者通力合作能夠極大推進(jìn)藥物研發(fā)的進(jìn)程。
但目前這種高利率環(huán)境卻讓初創(chuàng)企業(yè)舉步維艱,,因?yàn)樵谘邪l(fā)出藥物后,,往往要等好幾年才可以從產(chǎn)品銷售中獲得收入。由于高利率會(huì)帶來更高的成本,,大型制藥公司的并購意愿也會(huì)降低,。
2021年,有111家生物技術(shù)公司在美國進(jìn)行了IPO,,而到了2023年,,只有20家進(jìn)行了IPO。與此同時(shí),,生物技術(shù)公司合并或倒閉的壓力也在增加,。據(jù)安永(EY)統(tǒng)計(jì),半數(shù)生物技術(shù)公司的資金不足以維持18個(gè)月的運(yùn)營,。要想保持生物技術(shù)公司的創(chuàng)新動(dòng)力,,關(guān)鍵是要?jiǎng)?chuàng)造一個(gè)具有吸引力的環(huán)境,。
采用全新臨床試驗(yàn)?zāi)J剑A得患者信任
通過采用分散式的臨床試驗(yàn)策略,,我們能夠讓世界不同地區(qū)的患者都參與到試驗(yàn)之中,,從而提高患者對(duì)新研藥物的信任度。此外,,在進(jìn)行設(shè)計(jì)時(shí),,要考慮到哪類患者群體最有可能從中受益,尤其是那些未得到充分服務(wù)的患者,,使其具有適當(dāng)?shù)拇硇?,還要聽取這些患者的意見,這樣可以讓患者更容易接受新推出的療法,。
在針對(duì)上述各項(xiàng)問題進(jìn)行決策和行動(dòng)時(shí),,我們要小心謹(jǐn)慎,還要權(quán)衡利弊,,這樣才能充分推動(dòng)新的創(chuàng)新,、見解和工具產(chǎn)生最大的作用。通過加強(qiáng)與不同利益相關(guān)者的合作,,找到未知領(lǐng)域中的問題,,并制定相應(yīng)的解決方案,我們可以進(jìn)一步加快藥物發(fā)現(xiàn)速度,。(財(cái)富中文網(wǎng))
韓保羅(Paul Hudson) 是賽諾菲公司首席執(zhí)行官,。
Fortune.com上發(fā)表的評(píng)論文章中表達(dá)的觀點(diǎn),僅代表作者本人的觀點(diǎn),,不代表《財(cái)富》雜志的觀點(diǎn)和立場(chǎng),。
譯者:梁宇
審校:夏林
在這樣一個(gè)經(jīng)濟(jì)充滿不確定性、利率高企,、人才短缺,、資本成本不斷上升的時(shí)代,人工智能(AI)已經(jīng)成為許多行業(yè),、政府部門和學(xué)術(shù)機(jī)構(gòu)最關(guān)心的話題,。雖然還有很多問題亟待醫(yī)療保健行業(yè)解決,但我們有理由相信,,隨著人工智能技術(shù)的不斷發(fā)展,,藥物發(fā)現(xiàn)的黃金時(shí)代即將到來,而這將從根本上改變醫(yī)學(xué)領(lǐng)域的整體面貌,。
人工智能已經(jīng)開始在制藥研發(fā)(R&D)領(lǐng)域發(fā)揮作用,。在人工智能與數(shù)據(jù)分析的加持之下,業(yè)界實(shí)現(xiàn)了許多突破,比如現(xiàn)在我們已經(jīng)能夠預(yù)測(cè)患者反應(yīng),,臨床試驗(yàn)的成功率也有所提升,,并且已經(jīng)開始為患者確定個(gè)性化的治療方案。借助人工智能,,我們解決了許多技術(shù)難題,,解鎖了許多以前“無藥可及”的靶點(diǎn),為目前沒有治療選擇的患者帶來新的治療方法,。
在賽諾菲(Sanofi)的藥物發(fā)現(xiàn)和開發(fā)工作中,,人工智能技術(shù)已經(jīng)開始發(fā)揮重要作用。在小分子藥物發(fā)現(xiàn)方面,,我們關(guān)鍵人工智能模型的預(yù)測(cè)準(zhǔn)確率已經(jīng)達(dá)到80%以上,,而且通過主動(dòng)學(xué)習(xí),這些模型的準(zhǔn)確率還在不斷提高,。我們?cè)诖_定疾病靶點(diǎn)時(shí),,有90%借助了單細(xì)胞基因組學(xué)方面的知識(shí),,在推進(jìn)小分子項(xiàng)目時(shí),,有75%借助了人工智能與機(jī)器學(xué)習(xí)(ML)化合物設(shè)計(jì)。我們還創(chuàng)造了虛擬患者,,來推動(dòng)硅基臨床試驗(yàn),,最后,我們利用基于基因組學(xué)的精準(zhǔn)醫(yī)療技術(shù),,協(xié)助開展患者分層工作,。
我們使用先進(jìn)的主動(dòng)學(xué)習(xí)方法,持續(xù)改進(jìn)人工智能模型訓(xùn)練方式,,不斷減少模型訓(xùn)練所需的數(shù)據(jù),。人工智能學(xué)習(xí)凸顯出了關(guān)鍵結(jié)構(gòu)要素在引導(dǎo)設(shè)計(jì)周期方面的作用,可以縮短設(shè)計(jì)周期,,降低設(shè)計(jì)成本,,并提高設(shè)計(jì)出新分子的成功率。我們正在將臨床試驗(yàn)的數(shù)量增加50%,,根據(jù)現(xiàn)有數(shù)據(jù),,我們?cè)谘兴幬锏膬r(jià)值在2019年至2023年間翻了兩番。
我們始終堅(jiān)持與創(chuàng)新生態(tài)保持聯(lián)系,,采取“無邊界”藥物研發(fā)戰(zhàn)略,。我們25%的項(xiàng)目要與合作伙伴合作完成,按照每種臨床候選藥物所花費(fèi)的資金來衡量,,這種做法使我們的研究效率提高了一倍,,并讓我們能夠達(dá)到首次人體實(shí)驗(yàn)階段的藥物數(shù)量增加了一倍。
此外,我們的運(yùn)作方式也在發(fā)生深刻變化,?!皼Q策”不再是一種年度回顧性報(bào)告工作,而是變?yōu)榱藙?dòng)態(tài)的前瞻性決策方法,,將戰(zhàn)略選擇與業(yè)務(wù)決策聯(lián)系起來,,努力強(qiáng)化我們的反饋路徑。
我們現(xiàn)在顯然已經(jīng)站到了醫(yī)學(xué)發(fā)現(xiàn)大發(fā)展的十字路口,,但是,,制造企業(yè)要想充分發(fā)揮人工智能的優(yōu)勢(shì),充分利用其潛力,,我們還要克服若干具有深遠(yuǎn)影響的難題,。
監(jiān)管問題
不同地區(qū)在監(jiān)管方面存在差異,這種差異會(huì)限制人工智能的應(yīng)用領(lǐng)域和標(biāo)準(zhǔn),,同時(shí)還會(huì)對(duì)“高風(fēng)險(xiǎn)應(yīng)用”的定義產(chǎn)生影響,。
對(duì)數(shù)據(jù)質(zhì)量、安全性,、隱私和可信度的擔(dān)憂可能會(huì)減緩人工智能技術(shù)的應(yīng)用速度?,F(xiàn)在已經(jīng)出現(xiàn)了一些以幫助企業(yè)實(shí)現(xiàn)自我監(jiān)管為目的的聯(lián)盟和組織。
隨著許多企業(yè)開始全面應(yīng)用人工智能技術(shù),,防止漏洞出現(xiàn)就成了相關(guān)企業(yè)面臨的重要任務(wù),,而要達(dá)成這一目標(biāo),就必須打造強(qiáng)大的數(shù)據(jù)基礎(chǔ)和治理機(jī)制,。
限價(jià)的意外影響
新推出的定價(jià)政策可能會(huì)帶來一些意想不到的后果,,甚至可能降低投資人對(duì)一些很有希望的候選研發(fā)項(xiàng)目的投資意愿。例如,,《通脹削減法案》(Inflation Reduction Act)里就包含一些人口中所謂的“藥丸懲罰”條款,,相關(guān)條款規(guī)定,在小分子藥物獲批后9年,、生物制劑獲批后13年,,政府能夠?qū)π卵兴幬镞M(jìn)行限價(jià)??梢哉f,,這種規(guī)定從根上打消了藥企為老藥尋求新突破和新用途的動(dòng)力。還可能導(dǎo)致藥企加大對(duì)生物制劑的投資,,減少對(duì)小分子藥物的投資,。
生物制劑和小分子藥物具有同等價(jià)值。小分子藥物可以口服,,對(duì)許多患者而言更為方便,,在許多疾病的治療上也發(fā)揮著至關(guān)重要的作用,。
生物技術(shù)初創(chuàng)企業(yè)獲得資金的途徑
生物技術(shù)初創(chuàng)企業(yè)擁有良好的創(chuàng)新環(huán)境,是大型制藥公司研發(fā)工作的有益補(bǔ)充,。二者通力合作能夠極大推進(jìn)藥物研發(fā)的進(jìn)程,。
但目前這種高利率環(huán)境卻讓初創(chuàng)企業(yè)舉步維艱,因?yàn)樵谘邪l(fā)出藥物后,,往往要等好幾年才可以從產(chǎn)品銷售中獲得收入,。由于高利率會(huì)帶來更高的成本,大型制藥公司的并購意愿也會(huì)降低,。
2021年,,有111家生物技術(shù)公司在美國進(jìn)行了IPO,而到了2023年,,只有20家進(jìn)行了IPO,。與此同時(shí),生物技術(shù)公司合并或倒閉的壓力也在增加,。據(jù)安永(EY)統(tǒng)計(jì),,半數(shù)生物技術(shù)公司的資金不足以維持18個(gè)月的運(yùn)營。要想保持生物技術(shù)公司的創(chuàng)新動(dòng)力,,關(guān)鍵是要?jiǎng)?chuàng)造一個(gè)具有吸引力的環(huán)境,。
采用全新臨床試驗(yàn)?zāi)J剑A得患者信任
通過采用分散式的臨床試驗(yàn)策略,,我們能夠讓世界不同地區(qū)的患者都參與到試驗(yàn)之中,,從而提高患者對(duì)新研藥物的信任度,。此外,,在進(jìn)行設(shè)計(jì)時(shí),,要考慮到哪類患者群體最有可能從中受益,,尤其是那些未得到充分服務(wù)的患者,使其具有適當(dāng)?shù)拇硇?,還要聽取這些患者的意見,,這樣可以讓患者更容易接受新推出的療法。
在針對(duì)上述各項(xiàng)問題進(jìn)行決策和行動(dòng)時(shí),,我們要小心謹(jǐn)慎,,還要權(quán)衡利弊,這樣才能充分推動(dòng)新的創(chuàng)新,、見解和工具產(chǎn)生最大的作用,。通過加強(qiáng)與不同利益相關(guān)者的合作,,找到未知領(lǐng)域中的問題,,并制定相應(yīng)的解決方案,,我們可以進(jìn)一步加快藥物發(fā)現(xiàn)速度,。(財(cái)富中文網(wǎng))
韓保羅(Paul Hudson) 是賽諾菲公司首席執(zhí)行官,。
Fortune.com上發(fā)表的評(píng)論文章中表達(dá)的觀點(diǎn),僅代表作者本人的觀點(diǎn),,不代表《財(cái)富》雜志的觀點(diǎn)和立場(chǎng),。
譯者:梁宇
審校:夏林
Amidst economic uncertainty, high interest rates, talent shortages, and rising capital costs, artificial intelligence (AI) is top of mind for many industries, governments, and academic institutions. While there is a long list of challenges that the health care sector must overcome, there is reason to believe that through AI, we are on the cusp of a great era of discovery that could fundamentally change the field of medicine.
In pharmaceutical research and development (R&D), AI is already delivering on its potential. AI and data analytics are driving breakthroughs that enable us to predict patient responses, increase the likelihood of clinical trial success, and determine individualized treatment plans for patients. With AI, we are breaking new barriers to unlock previously undruggable targets and bring forward new therapies for patients who currently have no treatment options.
At Sanofi, leveraging AI to empower drug discovery and development is having a major impact. Our key AI models in small-molecule drug discovery are achieving more than 80% prediction accuracy–and they are constantly improving with the use of active learning. Ninety percent of our disease targets are credentialed using single-cell genomics and 75% of small-molecule projects are enabled by AI and machine learning (ML) compound design. We then create virtual patients to drive in silico clinical trials and, finally, genomics-based precision medicine will help us achieve patient stratification.
We are using advanced active learning approaches, improving AI model training, and requiring less data to train our models. AI learnings are highlighting key structural elements to guide design cycles, making them shorter and cheaper, and resulting in higher new molecular success rates. We are increasing the number of clinical trials by 50% and, to date, have quadrupled our pipeline value between 2019 and 2023.
We are in constant contact with the innovation ecosystem, adopting a drug discovery “without borders” strategy. Twenty-five percent of our projects entail working with partners, which has doubled research productivity as measured by dollars spent per clinical candidate and doubled our first-in-human entries.
Additionally, the way we operate is being profoundly altered. Decisions have shifted from an annual retrospective reporting capability to a dynamic prospective decision intelligence approach, linking strategic choices with operational decisions and seeking to enhance our feedback loop.
It’s clear that we stand at the crossroads of a great expansion in medical discoveries, but to take full advantage of AI, there are several challenges that will greatly impact the pharma industry’s ability to unlock potential.
AI regulation
Regional differences in regulation will guide restrictions on where AI can be employed, standards, and what constitutes high-risk applications.
Concerns about data quality, security, privacy, and trustworthiness have all threatened to slow the uptake of AI. Alliances and organizations are emerging to help companies self-regulate.
Strong data foundations and governance will be critical to prevent vulnerabilities as many companies move to operationalize AI across their enterprises.
Unintended effects of pricing restrictions
The unintended consequences of new pricing policies could diminish investment in promising R&D candidates. For example, the Inflation Reduction Act contains what some have called a “pill penalty” as it establishes price setting after nine years for small-molecule drugs compared with 13 years for biologics. It basically eliminates incentives for pursuing new breakthroughs and uses for older medicines. The result could be greater investment in biologics and less investment in small-molecule medicines.
Both biologics and small molecules are equally valuable. Small molecules can be administered orally, making them more convenient for many patients, and they also are critical to treating many diseases.
Access to capital for biotech startups
The biotech startup environment is a rich source of innovation that complements large pharma R&D efforts. The synergy between the two spurs drug discovery.
However, startups struggle in a high-interest rate environment as revenue from product sales are often years away. Higher rates also diminish large pharma M&A intentions as costs rise.
In 2021, 111 biotechs went ahead with IPOs in the U.S. In 2023, only 20 had IPOs. At the same time, there were increased pressures toward biotechs merging or going out of business. According to EY, half of biotechs do not have the cash needed to sustain operations for more than 18 months. Creating an attractive environment for biotech is key to maintaining power in the R&D innovation machine.
Building trust with new models for clinical trial design
Patient trust benefits from decentralized clinical trial strategies that allow those in diverse regions of the world to participate. This, coupled with designs that take into consideration the representation of the patient population most likely to benefit, especially underserved patients, and garnering insights from these patients, can create greater patient acceptance of novel therapies.
Decisions and actions on each of the above will need to be taken carefully, navigating trade-offs to ensure we fully drive the strongest impact from new innovations, insights, and tools. By increasing collaboration with diverse stakeholders to identify roadblocks and formulate solutions in these uncharted territories, we can drive faster discoveries.
Paul Hudson is the CEO of Sanofi.
The opinions expressed in Fortune.com commentary pieces are solely the views of their authors and do not necessarily reflect the opinions and beliefs of Fortune.